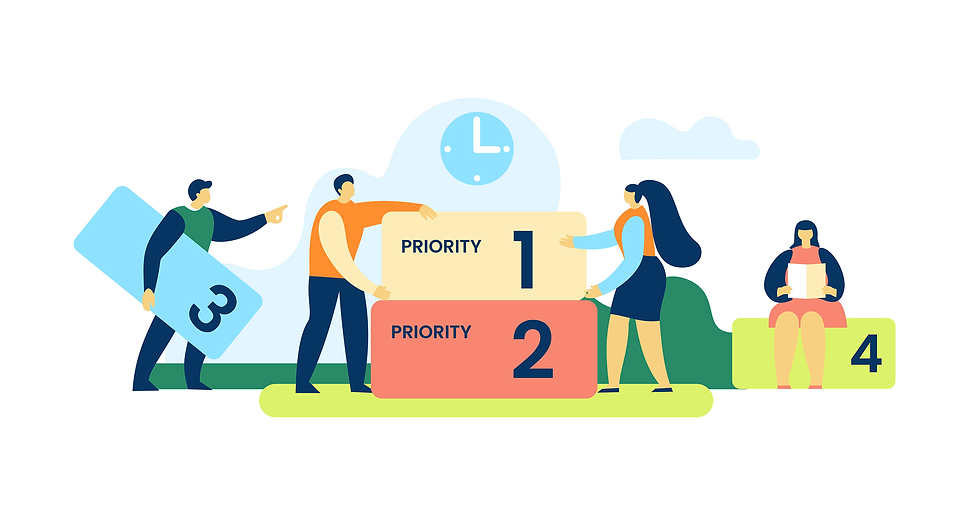
In the fast-paced world of product development, prioritization is a constant battle. With limited resources and an ever-growing backlog, product managers face the daunting task of deciding which features to build first. Traditionally, this has been done through user stories and stakeholder consensus, but these methods often suffer from subjectivity and lack of data-driven insights.
Fortunately, the rise of Artificial Intelligence (AI) and Machine Learning (ML) offers a powerful new approach to backlog prioritization. By leveraging these advanced technologies, product managers can move beyond user stories and make informed decisions based on objective data and predictive analytics.
The Problem with User Stories:
While user stories are a valuable tool for capturing user needs, they often lack the quantitative data needed for effective prioritization. Additionally, user stories can be biased towards vocal users and stakeholders, neglecting the needs of the silent majority. This can lead to the development of features that fail to resonate with the target audience and ultimately, deliver limited value.
The Power of AI and ML:
AI and ML offer a solution to these challenges by providing the ability to analyze vast amounts of data and extract actionable insights. By incorporating AI and ML into the backlog prioritization process, product managers can:
Predict user engagement and feature adoption: AI models can analyze user behavior, including click-through rates, feature usage, and user feedback, to predict which features are likely to be most successful.
Identify high-impact opportunities: ML algorithms can identify patterns and trends in user data, highlighting hidden opportunities and potential pain points that might otherwise be missed.
Prioritize the backlog dynamically: AI and ML models can be continuously updated with new data, allowing for real-time adjustments to the backlog based on changing user needs and market conditions.
Technical Implementation:
Several AI and ML tools are readily available to assist product managers with backlog prioritization. Here are a few examples:
Google Vertex AI: This platform provides pre-built machine learning models for user segmentation, churn prediction, and anomaly detection.
Amazon SageMaker: This platform allows developers to build custom machine learning models for specific business needs, including feature prioritization.
PredictiveHQ: This platform offers a suite of AI-powered tools specifically designed for product management, including backlog prioritization and user segmentation.
Integration with Agile Development:
AI and ML-powered backlog prioritization seamlessly integrates with existing agile development methodologies. By incorporating these tools into the sprint planning process, product managers can ensure that teams are working on the most impactful features first, leading to faster time to market and increased user satisfaction.
The Future of Backlog Prioritization:
The use of AI and ML in product management is still in its early stages, but the potential is immense. By leveraging these powerful technologies, product managers can make more informed decisions, build better products, and ultimately, achieve greater success in the marketplace.
Start exploring these innovative tools today and see how they can transform your product development process.
Comments